Global Manufacturer Lifts Production Line Productivity After Data-Led Product Testing Modernization
Our customer is a leading manufacturer of equipment and value-added services integral to the manufacturing practices of semiconductors and microelectronic devices. The product in this case study is a closed-cycle helium refrigeration system, typically used in laboratories for the direct cooling of experiments.
When our client began looking for ways to streamline manufacturing practices, they focused on the refrigeration testing process. It was a solid business decision as their refrigeration units are used in a variety of commercial and research applications, so increasing product output would give them a competitive advantage.
The plan was to improve the refrigeration testing process using advanced data technology solutions. Intelligent data capture and data analysis of the testing process plus machine learning models shortened the refiguration testing time and sped up product production.
The refrigerator testing process — before the project
Our client designed their refrigerator testing method 20 years ago. It was time for an upgrade.
The refrigerator units are tested in custom-designed stands, so only a limited number can be tested at one time. Testing each piece of equipment can take up to four hours.
The testing relied on static standard parameters and metrics — not actual data captured from the machines. In addition:
- The testing process acceptance criteria, metrics, and limitations were based on partial data and educated guesses.
- The process lacked data logic for many of the rules and decisions in the testing steps.
- Only a few engineers fully understood the testing process. Technical manuals existed, but the testing process itself wasn't documented.
They wanted to fix the testing methods and create advanced analytics to predict potential refrigerator equipment failures.
They had limited success with partners in the past and wanted to find a company with experience in manufacturing. They chose SoftServe because of our reputation for implementing successful data analysis and prediction solutions for manufacturing companies.
A project is only as successful as the quality of its data
Data was collected throughout the manufacturing process. However, the refrigerator data was scattered among many databases, files, and printouts. We knew of at least ten data-storing systems, and each needed to be integrated.
Finding refrigerator equipment history data, an essential part of building predictive analytics, took a lot of effort. Their data management problems had to be improved before any analysis could begin.
SoftServe started the project by conducting an extensive data review to reveal data merging and reliability pain points in our client's ecosystem. Although time-consuming, our team cleaned, contextualized, and merged the data required.
The success of any production optimization project relies on meticulous planning and diligent data organization. Here's a high-level glimpse of the project steps; the offsite work was often a trial-and-error process until we found the correct analysis and modeling criteria.
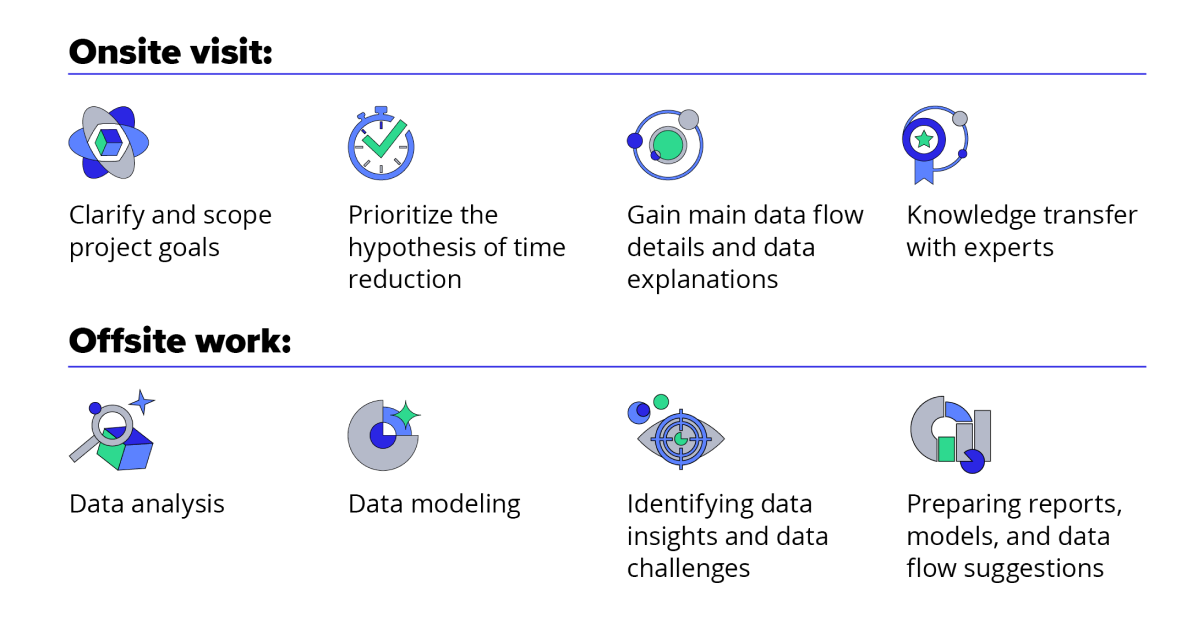
The refrigerator testing process now — a resounding success
Our client’s executives were pleased with the impressive and extensive project results. As we wanted you to fully understand the scope of improvements that occur when a data project is done right, we broke down the results and successes into three categories: data analysis, project outcomes, and graphical interfaces.
Data analysis
In our analysis, we explored reducing the refrigerator testing time using pass/fail predictions during the test and in the field. Valuable analysis during this process provided exceptional results, like:
- Discovering refrigeration unit retesting and reloading can increase testing productivity by 10%-15%.
- Eliminating unnecessary retesting of refrigerators saves at least 6% of the total time spent on testing.
- Building several machine learning models with a 67% prediction accuracy rate.
- Predicting correctly in 73% of cases whether the test will pass or fail in future stages.
- Realizing 10%-15% cost savings as a result of optimizing the testing flow.
- Designing new refrigerator stands based on data analysis saves even more testing time.
Project outcomes
The goal of this project was to improve the refrigeration testing process. Our client considered this the first step to becoming a data-driven company that uses applied data science techniques. The business intelligence and machine learning models created during this project put them on the right trajectory.
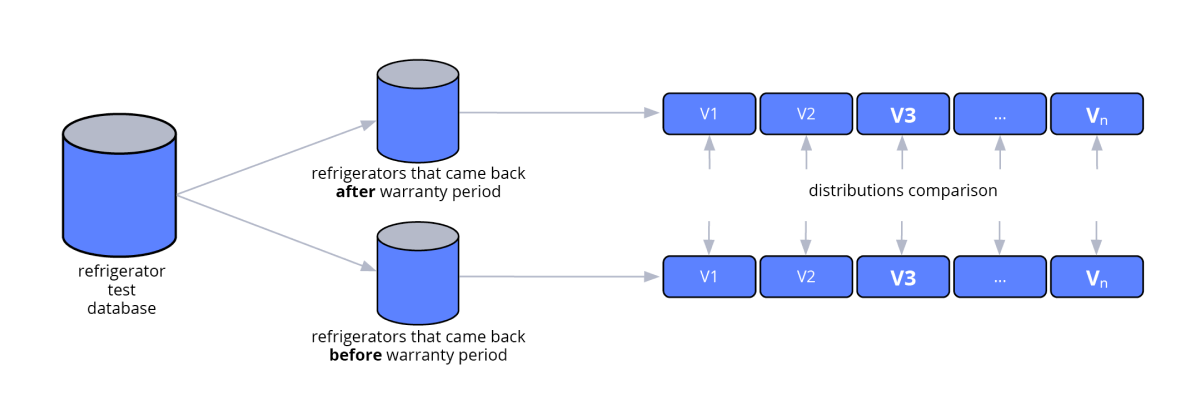
- The client noticed the difference between the behavior of refrigerators that reached their warranty and those that came back early.
- We delivered data and statistical proof of some suspicions from company engineers and pointed out a few other reasons for failures.
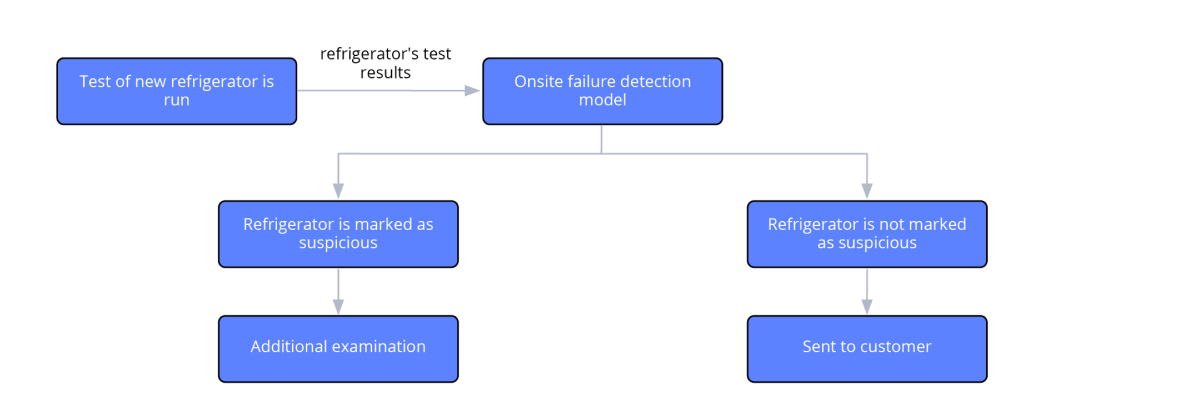
- Random forest models have been delivered separately for each of the refrigerator models.
- The top model for the subset reached a 0.85 ROC AUC score.
- The model could classify suspicious refrigerators that needed further examination to determine the likelihood of failure at the customer location.
We designed two models to test the data's predictive power. Could the data figure out at what future points the refrigerator would fail or not?
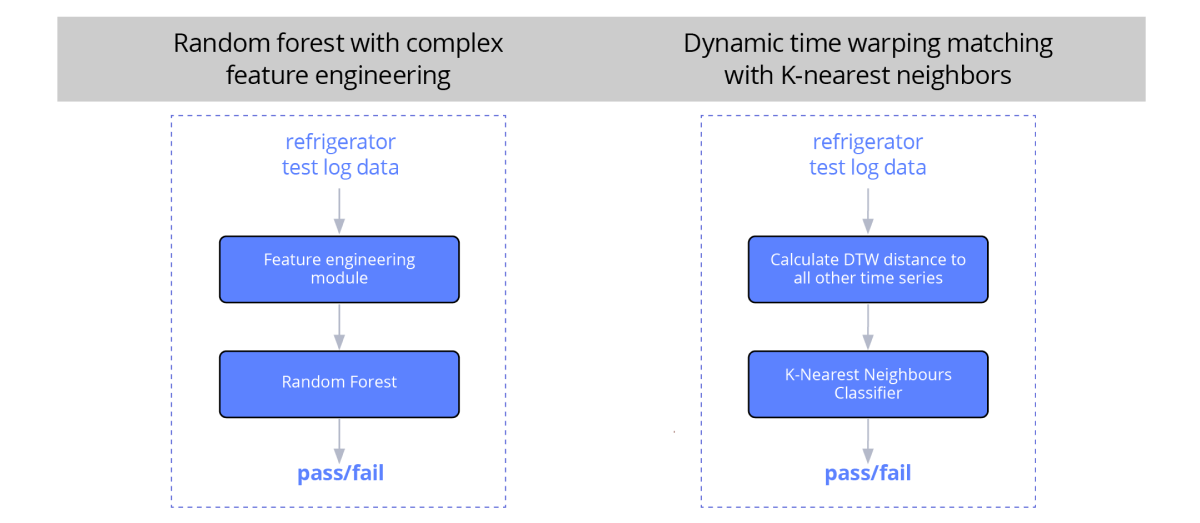
Graphical interfaces
At specific points in the project, the SoftServe team recognized the need for graphical interfaces to help with predictive modeling and testing.
We created several actionable graphs, including:
- A chart that represents the different sensor readings from each testing stage to depict how each refrigerator behaves. It provides an analysis of any deltas that could be significant.
- A model that searches for early failure indicators in the first half of testing. The failure is flagged, testing is halted, and the refrigerator is sent to diagnostics.
Also, we built a user interface for the testing process, which includes:
- An app to show predictions of whether a refrigerator test will fail.
- Important features of the predictions are visible and relate to the reason for each prediction.
- An option for engineers to provide feedback as to whether they agree with the prediction or not.
SoftServe not only modernized our client's refrigeration testing process, but we also improved the user experience. This project’s advanced data management practices have now been fully embraced by our client, forming a roadmap for upgrading their other manufacturing production lines.
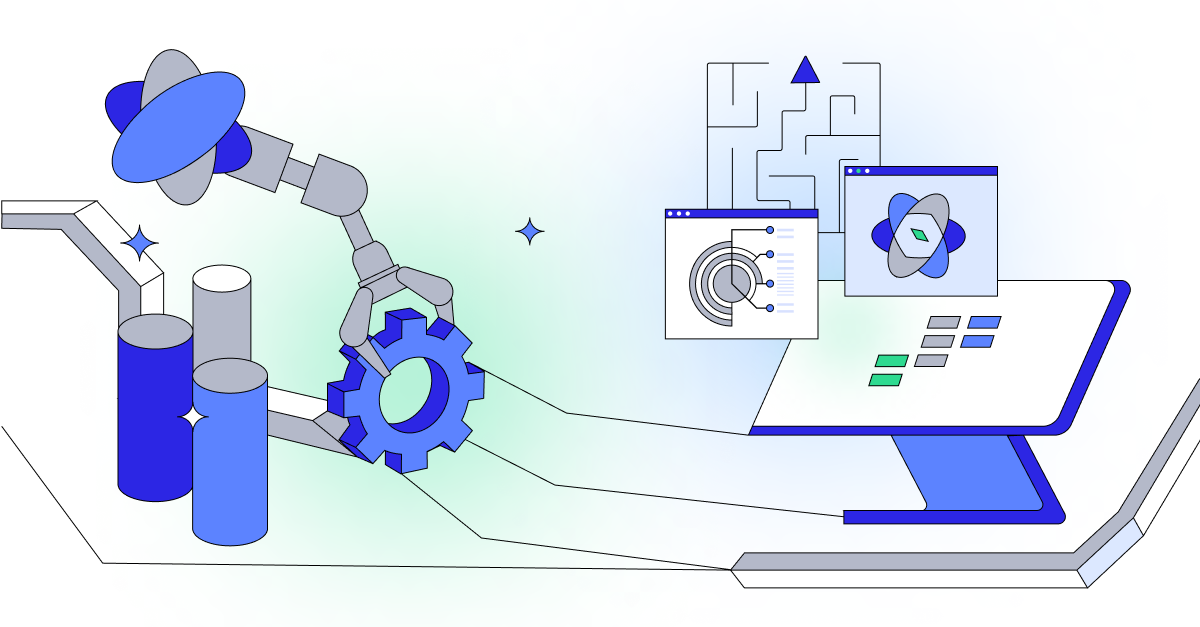
Need your data updated to keep up with evolving production demands? Discover how SoftServe’s Production Data Tower can help you transform your data into actionable insights.