By Andriy Bench, R&D Engineer
Technology offers many possible solutions for biometric identification to prevent fraud or unauthorized access. The global pandemic has created an urgent need for contactless biometric identification. SoftServe’s experts have been paying close attention to the “touchless” solutions that can potentially fulfill the need for non-contact in the new normal.
In this whitepaper, we’ll review different contactless solutions, including their progress and challenges.
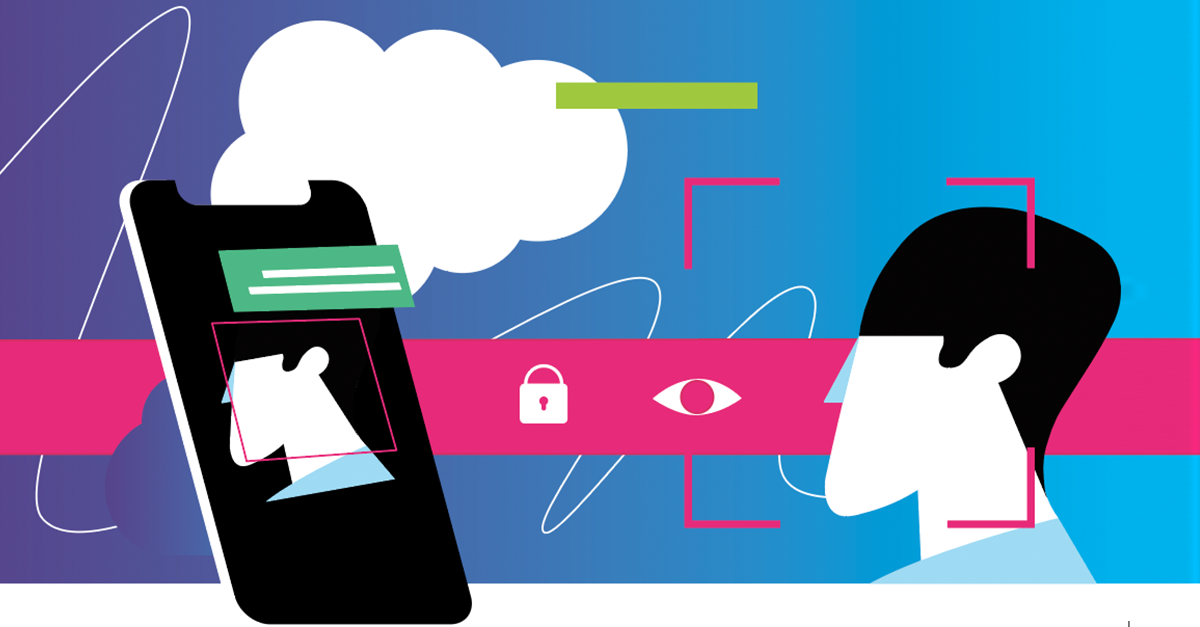
Facial Recognition
Face identification—a popular Face ID beta project solution used in modern smartphones—is suitable for touchless biometrics solutions. Unfortunately, during the pandemic period (with people wearing face masks that cover both nose and mouth), algorithms that detect facial landmarks are rendered non-functional.
Here's when the use of image recognition (IR) cameras comes into play.
IR band is divided into Near-Infrared (NIR), Short-Wave Infrared (SWIR), Mid-Wave Infrared (MWIR), and Long-Wave Infrared (LWIR) sub-bands. The first two are reflection dominant bands and are usually used for capturing face and iris images. NIR helps in low-light conditions, whereas SWIR is used in lower visibility instances.
MWIR and LWIR sub-bands are emission dominant and produce thermograms of an object. For the use of ML and AI algorithms, corresponding data sets have been created containing images obtained in different spectral bands:
- Carnegie Mellon University Hyperspectral Face Dataset (CMU-HSFD)
- The Hong Kong Polytechnic University Hyperspectral Face Database (HK PolyU – HSFD)
- UWA Hyperspectral Face Database (UWA-HSFD)
- CASIA HFB Database
- CASIA NIR-VIS 2.0 Database
Deep neural network (DNN) architectures have also been adapted in IR solutions. For example, GoogleNet was modified using the CASIA NIR data set to obtain NIRFaceNet.
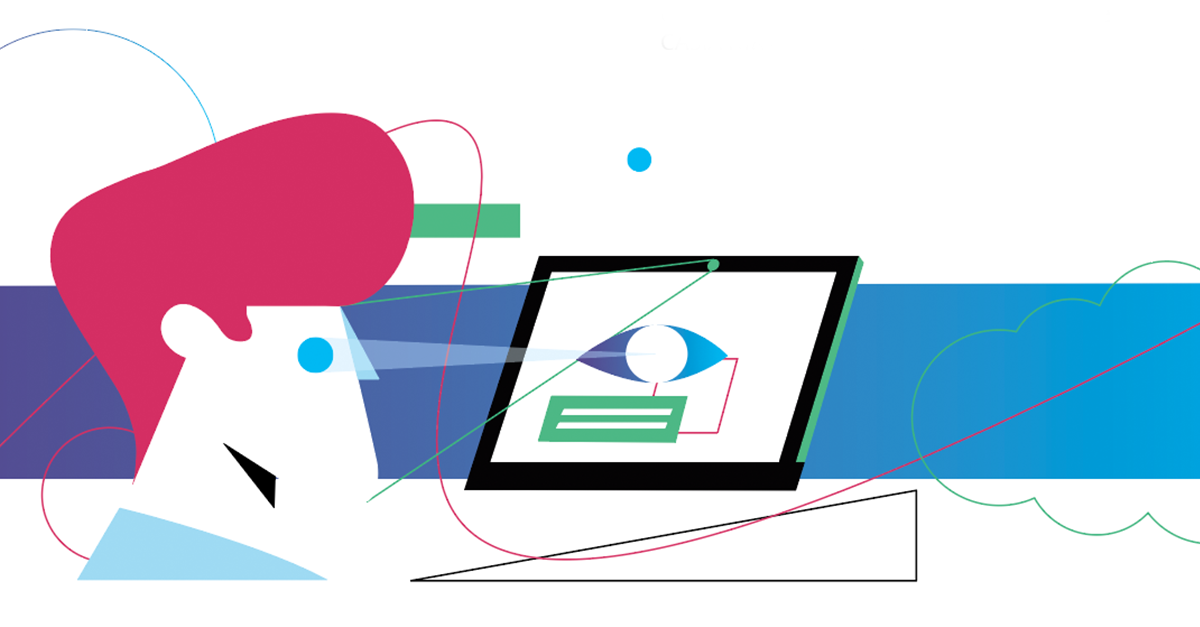
Iris Identification
Even when a person is wearing a mask, the periocular region of the face is usually visible, and iris identification is possible. Most commercial iris identification systems work in near-infrared range to break down the reflection patterns and variations in color.
Several public iris identification databases exist:
- IIT Delphi Iris Database
- CASIA-Iris-Thousand Database
- IIITD Multispectral Periocular Database
- ND-IRIS-0405 database (captured by LG2200 iris image camera)
- CrossSensor-Iris-2013 database (captured by both LG4000 and LG2200 camera)
- Visible Light Mobile Ocular Biometric Dataset (acquired by iPhone 5S, Samsung Note 4 and Oppo N1 mobile cameras in three different illumination conditions)
- Cross Sensor Iris and Periocular Database (captured by Sony Ericsson Xperia Arc, Apple iPhone 4, ThL W200 and Huawei U8510 with both frontal and rear cameras with and without flash and under different illumination conditions)
Additionally, new deep learning (DL) methods have been adopted for iris identification:
- The usage of VGG-Net (accuracy level about 99.4% on IIT Delhi Iris Database) and LightCNN29 networks for iris identification
- The creation of DeepIrisNet –A, DeepIrisNet-B as well as the usage of traditional image processing approaches (Haar Wavelet - 96.6% accuracy, Log-Gabor Filter - 97.2% accuracy, Fusion - 97.4% accuracy, Elastic Graph Matching - 98% accuracy, texture+scattering features - 99.2% on IIT Delhi Iris Database)
Last year’s research in the field of iris identification used 3D models of iris (reconstruction from 2 photos) instead of 2D images to make identification more robust. Commercially available Iris recognition sensors were proposed by AOptix Technologies.
Ear Identification
Ear identification is used when a person is wearing a mask or respirator. Recognition challenges arise because ears are usually captured under different angles and lighting, often with part of the ear covered by accessories.
The first ear Identification methods were built on a constrained image database, using one camera angle, and in single lighting conditions. Usually, simple geometric, local (descriptor-based) image approaches, or a combination of both were used. Early solutions also sought to adapt well-known image classification architectures (VGG, ResNet, ImageNet) to ear recognition.
The most recent field testing uses different deep learning networks for ear identification. More sophisticated solutions were specially built for ear recognition through a three-stage process:
- Whole ear image is captured
- Different parts are captured to analyze different ear regions
- Data from first two is combined
Networks are usually trained and tested on Extended Annotated Web Ears (AWEx) or the Unconstrained Ear Recognition Challenge (UERC) data set. Original data sets are usually modified by combining ear images with images of generated ear accessories.
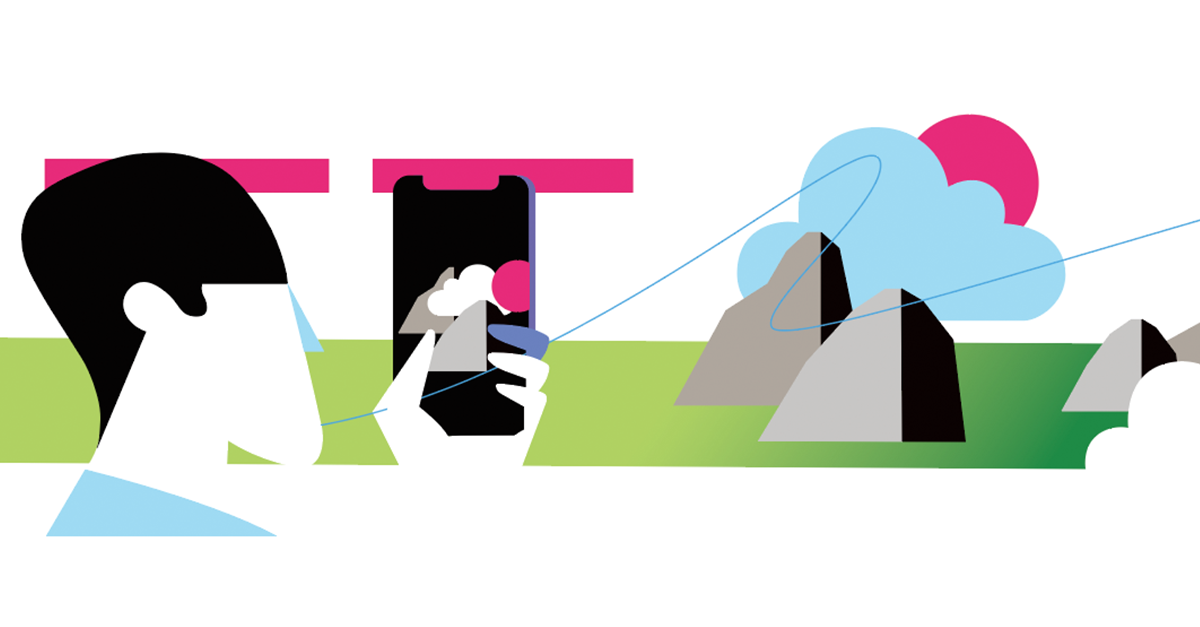
Gait Identification
Researchers claim that walking style is unique for every person which has resulted in different gait identification systems. Many systems use video from surveillance (or similar) cameras to analyze silhouettes or binarized video patterns.
There are also quick and cheap implementations reusing existing Wi-Fi infrastructure with CSI capabilities with sensors using new DL techniques for classification. More complex solutions require covering a floor with different sensors including pressure, light, capacitance, and EM noise.
Such approach is slower to be deployed. An additional advantage of gait identification is the possibility of recognizing human emotions simultaneously with identification.
Fingerprint Identification
Fingerprints have long been used as a method for biometrics identification and became the most popular solution for unlocking phones, laptops, and other smart devices. The main challenge during the pandemic, of course, is that most sensors require tight contact with a finger.
Fortunately, contactless fingerprint sensors have been intensely researched by scientists and developers over the last several years. Today, there is already a wide range of different contactless solutions on the market ranging from researchers/hobbyists' proposals of web camera use with an open CV library for processing, to state-of-the-art sensors and solutions that reuse smartphone cameras.
Some solutions have been certified by the United States’ Federal Bureau of Investigations (FBI) in response to hackers stealing fingerprints from high definition images placed in online media.
Contactless fingerprint identification allows us to improve hygiene, speed up access control, and identity processing in high traffic areas—but recognizing the many shapes and sizes poses a challenge. As long as individuals are encouraged to wear protective gloves in public, this technology will also be subject to pandemic-caused limitations.
Additional Non-Contact Methods
Additional solutions that have found their way to market include:
- Finger vein (popular in banking and financial applications)
- Retina identification (requires an eye in front of the sensor)
- Hand palm or dorsal palm
- Footprint (applied in places where people are usually without shoes: swimming pools, beaches, hospital beds)
- Voice (works for people in face masks as well as biosafety suits with additional recognition system setup)
Again, the current pandemic limits many of these options, but by combining several contactless methods it is possible overcome the temporary challenges created by social distancing and protective measures. Enterprises already or planning to leverage biometric security could gain a competitive advantage by exploring the right combination of traditional and contactless identification solutions.
Let’s talk about where you are in the biometric identification journey and how our experts can help you to accelerate your contactless security measures for the future.