AI Explainability and Adoption in Manufacturing
Accelerate AI adoption in your production facility with enhanced transparency and auditability
SUMMARY
![]() |
![]() |
![]() |
AI algorithms offer unbeatable accuracy and performance for automating many tasks, but non-technical stakeholders have difficulty understanding how AI works. |
This creates a perception of unreliability, which hinders companies’ adoption of AI algorithms and restricts them to classical methods, such as computer vision. |
Using explainable AI (XAI) techniques, technical stakeholders can help their business-side peers to validate the value-add of AI models and support their adoption. |
INTRODUCTION
You have probably encountered the problem of explainability in artificial intelligence (AI). In manufacturing as elsewhere, AI algorithms are a black box for many non-technical stakeholders. They lack the training to understand the inner workings of the models and their results. Because they must perform due diligence before supporting projects, that impenetrability blocks investment in promising AI initiatives.
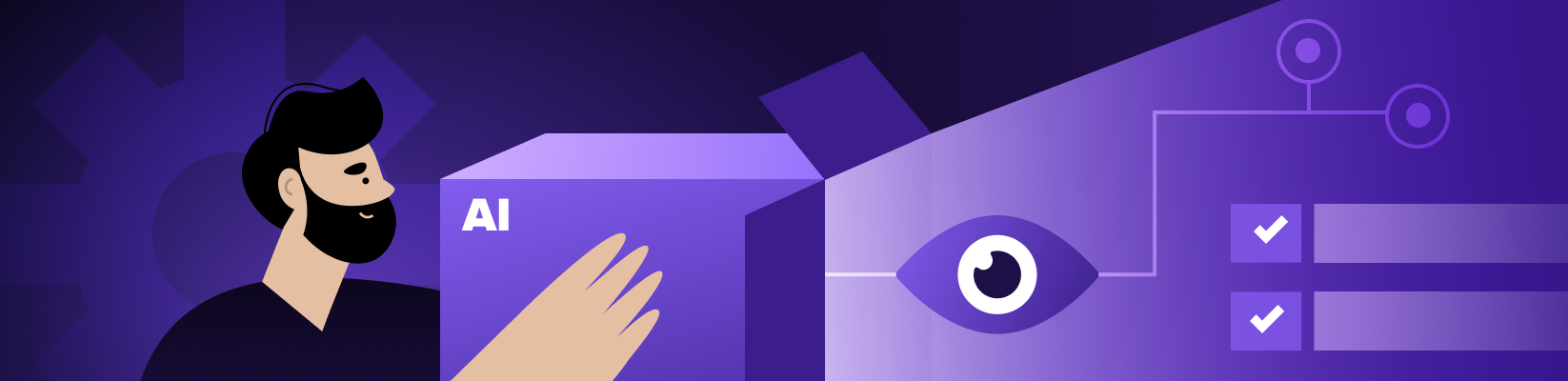
With explainable AI (XAI) techniques, you can solve this challenge. They enable you to build AI systems that provide clear and accessible explanations of their results. By incorporating XAI techniques into your AI projects, you make them transparent — and trustworthy. That gives all stakeholders the confidence they need to support AI projects. So, how exactly does XAI overcome the problem of explainability?
AI VS. CLASSICAL ALGORITHMS
Explainability is key to accelerating digitalization. In the transition to Industry 4.0, technology initiatives are only effective with broad backing and confidence. Stakeholders need to trust the advanced algorithms that promise to ensure quality, reduce human error, and increase production bandwidth.
At this point, you have two possible approaches to achieve these aims in industrial contexts:
![]() |
![]() |
|
Classical solutions |
AI algorithms |
|
|
|
While classical solutions — such as computer vision — are valuable, AI surpasses them in most metrics. But because AI is a black box for many non-technical stakeholders, they are often reticent to underwrite AI projects. That means that engineers must rely on classical solutions and their moderate performance.
Solving the tradeoff between accuracy and understanding is crucial to ensure that superior AI-based systems enjoy positive reception across stakeholders. Only then will you be able to take advantage of their greater effectiveness.
WHEN BLACK BOXES BLOCK IMPROVEMENTS
Consider the dilemma facing a group of engineers at a large industrial company. They had long relied on classical algorithms to identify and classify production errors during a quality assurance procedure. To boost quality across the board, the team sought to create a more accurate AI algorithm that could be used for multiple types of objects.
After working with the engineers to evaluate the status quo, we proposed an AI model to meet their goals. However, the engineering team had already conducted experiments with their own AI prior to our collaboration. Although the AI model improved classification accuracy by 12%, key stakeholders remained skeptical — and justifiably so.
Specifically, though the model worked well on one set of data during experimentation, it failed to generalize to new sets during production. While algorithms often require multiple iterations before achieving satisfactory results, the engineering team couldn’t adequately explain the model’s behavior. So, the stakeholders blocked its adoption.
ILLUMINATION THROUGH EXPLAINABILITY
To help the engineering team validate its AI strategy, we helped them develop XAI techniques to analyze their existing model. We used two main tools: Class Activation Maps (CAM) and SHAP values.

Class Activation Maps (CAM) |
SHapley Additive exPlanations (SHAP) Values |
|
To produce a heatmap tracing the portions of an image to which the AI algorithm was paying attention. |
To provide a visual representation of the factors that most influenced the AI algorithm’s decisions. |
After opening the black box together, we found that the existing model did indeed have a problem. It had targeted the wrong parts of the images and thus learned to focus on irrelevant features.
With that discovery in hand, our teams were able to develop an AI model with interpretable and correct results. In the end, the AI model achieved a 10% accuracy improvement compared to classical methods. More importantly, the engineers were able to explain the model's predictions and prove that the results were correct for all datasets.
With that insight, the non-technical stakeholders gained the visibility they needed to sign off on the project. The AI model was adopted.
BREAKING DOWN BIAS WITH XAI
With explainable AI you can give business-side stakeholders the confidence they need to support AI projects. That’s because you will be able to communicate your own enhanced understanding of the algorithm with the help of the visual and direct quantitative XAI metrics. That means more AI projects with better results — and improved performance across the board.
In the era of Industry 4.0, accelerating digitalization, and tight competition, manufacturers can’t afford to drag their feet on AI. But AI needs to be transparent, understandable, and trustworthy to be effective.
If you’re interested in using XAI techniques to advocate for powerful AI algorithms and improve your production processes, let’s talk.