At a recent Retail Hive conference in London, almost 100 retailers from all parts of the UK were discussing major challenges and ideas around artificial intelligence (AI) and machine learning (ML) implementation to support fulfillment, the supply chain, and the retail industry in general.
As a moderator at a series of roundtables and brainstorming sessions at this Retail Hive event, I’ve compiled the points below to save time, energy, and money during AI/ML project implementation.
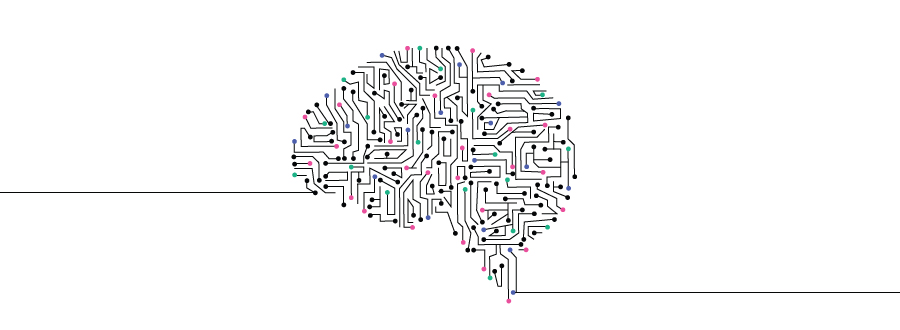
AI- and ML-based projects vs. traditional analytics
The retail industry is one of the most data-driven industries, and retailers have run analytics projects for decades. So, what has changed with new technologies like AI and ML?
It’s a good question. While analytics is a strong methodology, AI and ML are more powerful and can give more insights on a bigger amounts and variety of data.
But there is also a human and process aspect to consider. Previously, retail subject matter experts (SMEs) and business stakeholders gave a task to their analytics team to obtain specific analysis or statistics. Today, data science engineers are able to explore all of the combinations of data, using hundreds of AI/ML algorithms to get correlations and anomalies from the data on their own. They then get back to the business and explain these findings.
In other words, traditional analytics used to support a hypothesis and what is already known. AI and ML, by contrast, are tailored to provide yet unknown insights, opening new horizons for improving the business.
Main focus of AI/ML projects in retail
Our teams have spoken to dozens of the biggest retail companies about their AI and ML projects and areas of research.
It stands that demand prediction and forecasting, promotion and marketing campaign optimization, price optimization and personalization are the main areas of focus for these and many other companies.
With considerable customer demand for click-and-collect solutions and personalization, AI and ML systems allow for competition with retail giants like Amazon. They enable the same—or better—speed, impact, and efficiency in delivery, fulfillment, assortment planning, price optimization, and personalization.
An algorithm is only as good as its data
A great algorithm is impossible without a sufficient variety and volume of quality data.
Quality is an obvious factor. Improving poorly structured, inaccurate, or contradictory data will take time, leaving little room for developing the algorithm itself. And sufficient volume is required for the maximum efficiency of AI/ML algorithms. For example, a history of any 10 transactions results in limited accuracy, while algorithms with millions of transactions show maximum efficiency.
Variety of data is also important. Business leaders should make sure that the algorithm covers all influence factors; not just the obvious transactional and weather data, but something that goes above and beyond. Retailers should consider the most unusual data sources available from customers for a complete picture of customer needs.
Look at existing data from a different angle
Even when considering “obvious” data sources, try to think out of the box and look at traditional data from another angle. Take weather reports and forecasts: go beyond temperature to consider factors such as humidity, cloud coverage, wind speed, and direction.
Looking for more information on the best practices for AI and ML projects within retail? Check out our upcoming white paper, “AI + ML in Retail: Best Practices.”