Seismic techniques play a significant role in oil & gas exploration by covering large areas and unveiling subsurface structures with more precision. Seismic acquisition methods, however, remain less than ideal as we begin a new decade in 2020. A primary contributor to ongoing seismic data (SD) challenges is noise—or any unwanted features in the data.
Examples of random noise include wave action in a marine environment, wind and vehicle traffic in a land environment, and electronic instrument noise in both environments (as well as cable vibrations and drilling operations near seismic acquisition).
Generally, this noise provides no information about the subsurface, rendering a seismic survey useless and a waste of effort. Consider the following data examples with and without noise:
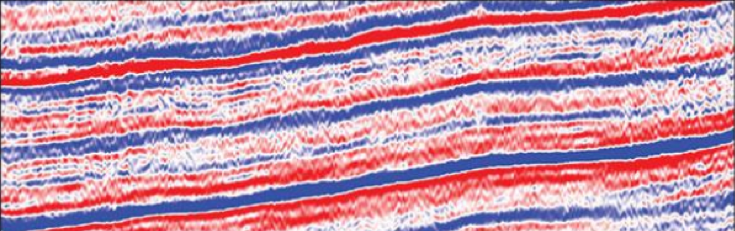
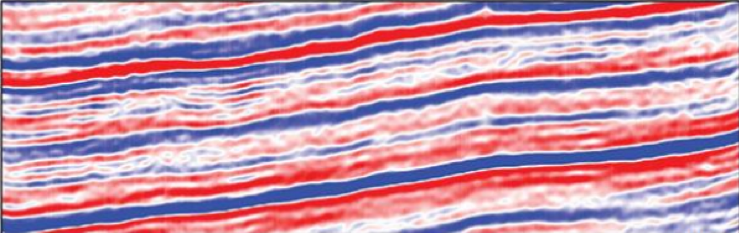
Top image. Seismic Data with subtle cross-cutting noise
Bottom image. Same data after noise filtering
Random, coherent, and decay
There are three types of seismic data noise to touch on:
Generally, random noise is an easy target to recognize and suppress with existing filters.
Coherent noise is more complex and addresses undesirable seismic energy that shows a consistent phase from trace-to-trace. For example: acquisition footprint, shallow refractions, and multiples.
Coherent noise is more challenging to overcome than random noise, and may result in coherent artifacts on seismic attribute displays that can mask features of interpretation interest.
Finally, signal decay can occur under certain geological conditions such as the decay of a signal under salt deposits which are one of the indicators (and prerequisites) for the formation of oil or gas reservoirs—so, the maximum possible signal quality in these regions is extremely important.
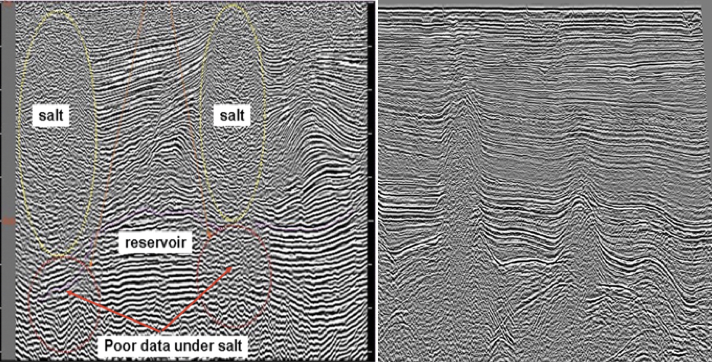
To increase the quality of the output data, SoftServe is building a solution to clean up SD noise.
Remove SD noise
To solve SD noise challenges, we’ve based our solution development on a deep learning (DL) neural network model called Super-Resolution Generative Adversarial Network (SRGAN). This intelligent model restores high-resolution images from low-resolution images.
Here’s how the simple two-step process works:
- We remove high frequencies from the data.
- We use SRGAN to recreate all parts without noise.
Observe the significant improvement in detail in the following before and after de-noising example:
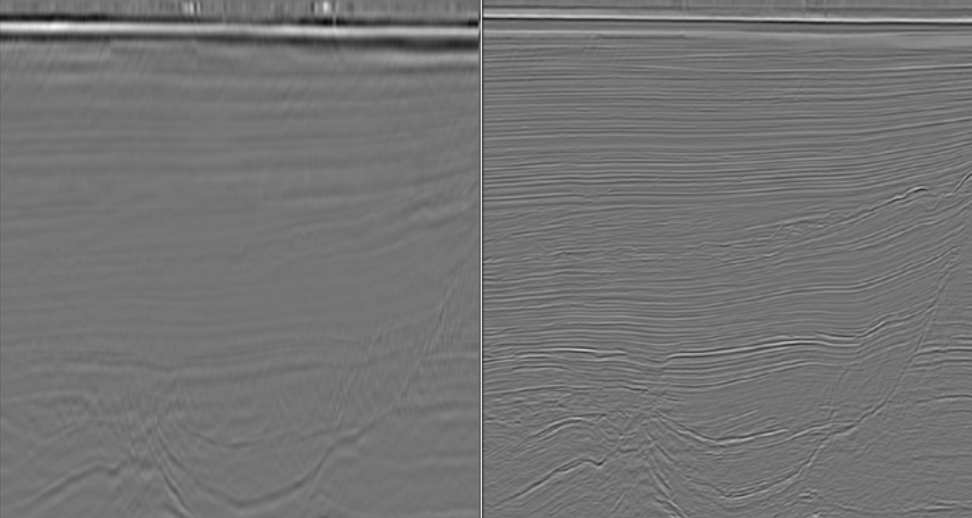
Data in-painting
Inevitably, there will also be gaps in seismic data. We’re addressing this challenge by developing a complimentary solution that leverages generative adversarial network (GAN)—a set of generative machine learning (ML) models.
GAN is used for predicting and generating new content and consists of two neural networks to predict and generate data by an existing dataset. NVIDIA uses this network to increase graphic quality, and our Data Science team learned how to fill gaps and broken parts of seismic data using a similar approach.
Here’s an example of our data in-painting solution in action:
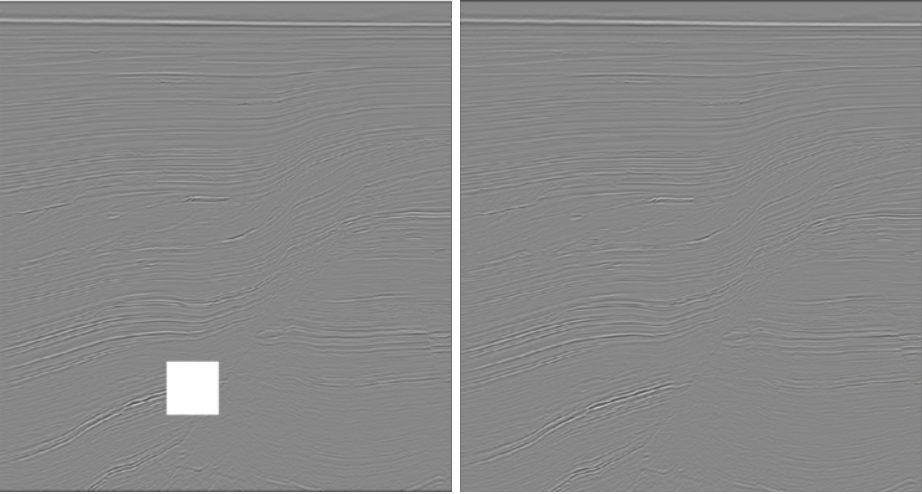
Seismic data noise detection and removal, as well as data in-painting are core techniques in SoftServe’s single, user-centric solution for full-cycle, seismic data processing.
This solution empowers a simplified process allowing users to:
- Upload files to a data lake
- Access processed data via an intuitive web interface or third-party tools integrations
- Review a seismic model of the sub-surface structural, depositional, and erosional features (faults, channels, horizons, salt bodies, etc.).
Read our latest whitepaper, ML FOR SEISMIC DATA PROCESSING to learn how our global team of AI/ML, big data, and DevOps experts empower our clients to reduce seismic data processing time from months to days, while eliminating errors for greater accuracy and efficiency in O&G exploration.