ISV and Digital Native Roadmap to Machine Learning Success
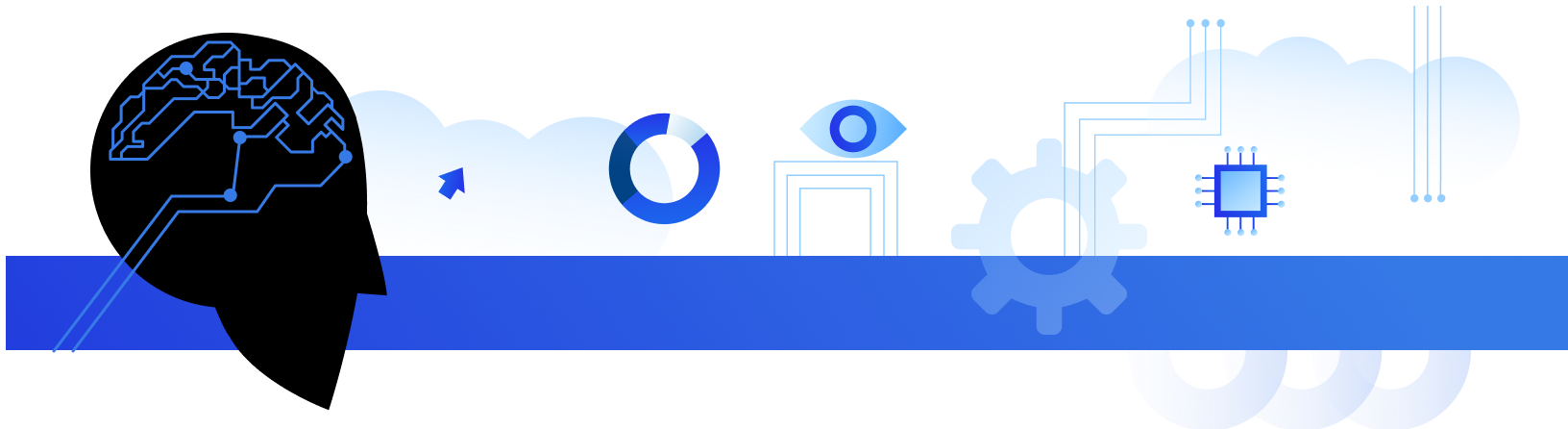
A short while ago, SoftServe published a blog article that answers the question: Why are artificial intelligence (AI) and machine learning (ML) projects not meeting the expectations of software company executives?
Recent research from SoftServe shows that software company executives believe AI/ML is imperative for them to compete and grow. The same study reports AI/ML initiatives fall short of their expectations (97%).
Why are expectations falling short? Because those organizations miss the genuine business value of AI/ML to drive business outcomes.
Now, in the following blog article (the first in a series of three), we explore how software companies — independent software vendors (ISVs) and digital native businesses (DNBs) — can move from AI/ML science experiments to monetized features that hit their business goals.
While focusing on SoftServe’s research (Real State of AI/ML Capabilities in Software/Digital Native Companies), most software companies — especially those in the business of brokering and publishing data — can receive help from the study’s recommendations.
Below is a review and elaboration of the six foundational recommendations SoftServe offers from the analysis of responses given by 600 technology executives and leaders. The analysis of those recommendations is complemented by SoftServe’s nearly 30 years of experience building products for software companies.
In follow-up blog articles, we’ll interview two Center of Excellence leaders from SoftServe about specific challenges software companies face implementing AI/ML-powered product features.
Here are SoftServe’s six foundational recommendations.
1. Strategic alignment
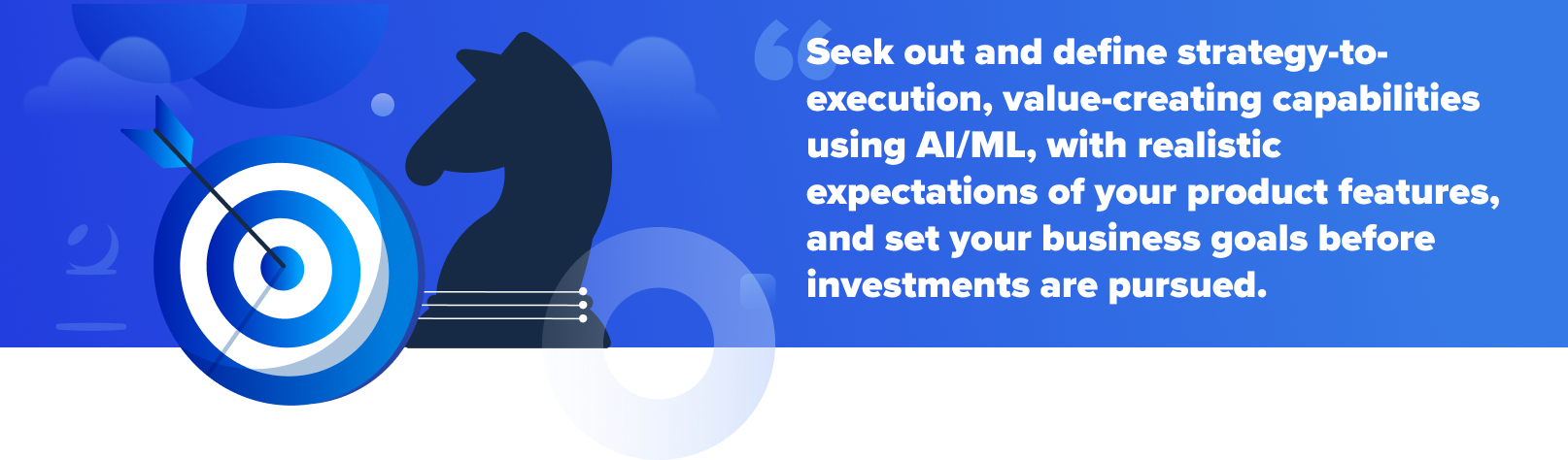
Software companies need to move from thinking of AI/ML as science experiments and ways to appease the curiosity of top tech talent. The mindset and culture of engineering and product teams must change from using these technologies as tools, like computer languages and cloud services. Some will have specialized skills in the tools, while others will focus on other tools.
A principal challenge found in SoftServe’s research is company leadership often doesn’t understand the business cases best served by these newer technologies. Software traditionally is a top-down and determinant activity; AI/ML requires thinking of statistical likelihoods. Finding the strategic value to both your business and your customers starts with understanding exactly how AI/ML is applied as a tool.
Education is key. Consider forming an AI/ML working group, either formally or virtually, with representatives from disciplines across your organization. This includes administrative functions (often, they are the best source of use cases) to explore use cases and theorize product features.
If your leadership doesn’t understand AI/ML, encourage them to read McKinsey’s An Executive's Guide to AI.
2. Product ownership
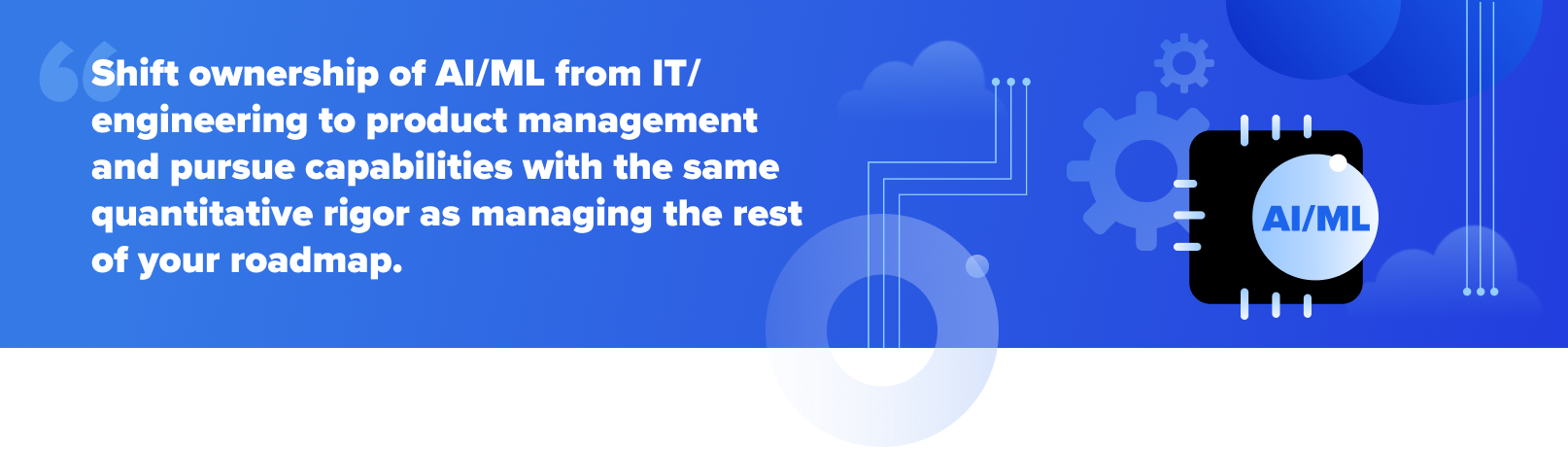
You must adopt and promulgate an attitude toward AI/ML technology that it’s just that: a technology. Like other technologies, success means appropriately applying the technology.
All experimentation with data, systems, and tools contributes to building skills and capabilities. It’s now time to apply those capabilities to product features, and product management typically leads with defining, prioritizing, and managing those features.
While you can focus on customer problems without regard to the technology, you don’t want to uncover problems to solve just because you have the right tools. Product managers need to understand how their tools help solve problems.
For example, product managers who are well-informed about microservices architecture will shape features and solutions suitable for implementation in microservices. Similarly, product managers must become well-informed about the what and why of AI/ML, but not necessarily the how.
Particular attention must be given to the most critical part of the AI/ML product feature equation: data. Product managers don’t need to be data scientists, but they must minimally understand the data available to them. Working groups and ideation meetings with customers and data scientists ultimately lead to pinpointing insights found in the data you’re collecting and inferences to apply AI/ML.
Lastly, product managers can’t sacrifice the quantitative rigor needed to manage feature roadmaps just because some of the features are statistical and emerge from the black box of an ML model. Focus on quantitatively figuring out whether those features lead to customer value, which brings you to the next recommendation.
3. Customer validation
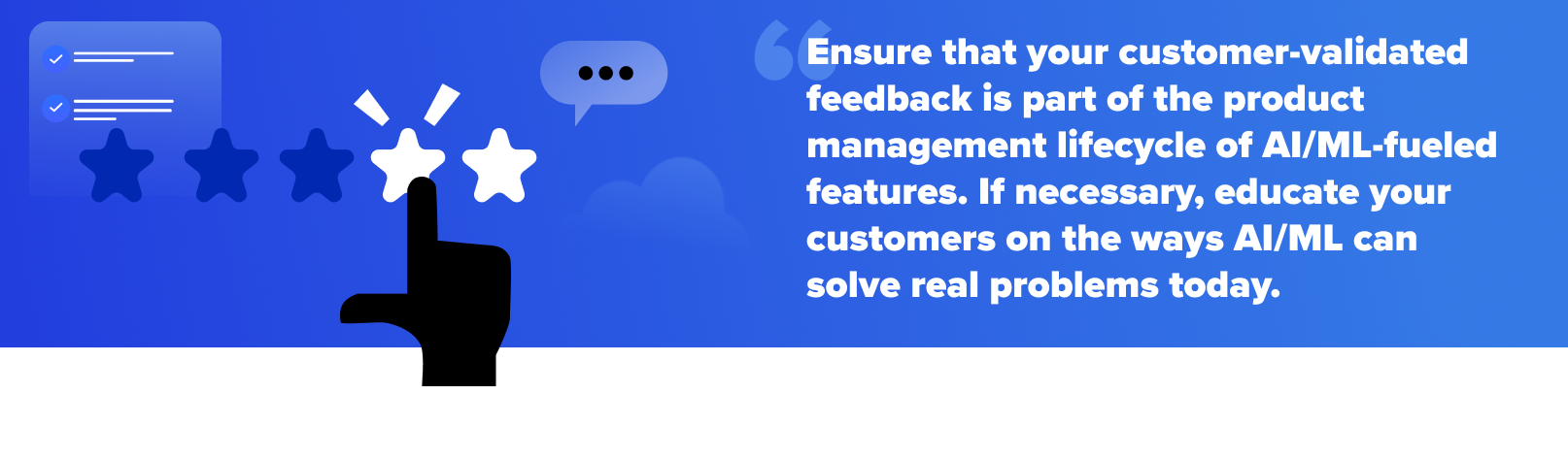
Product managers can’t bypass customer-validated feedback for product features, including AI/ML and even if there’s a black box effect. Some customer education is likely needed for more complex features. For example, getting them to trust how predictive models work: that is, education about your model’s technique and education in AI/ML in general.
For more visual feature applications — finding objects in imagery, for example — users tend to understand the statistical nature of the activity more naturally. After all, people identify objects in imagery with a certain amount of statistical accuracy.
What’s particularly critical in customer-validated feedback is when your underlying model requires training and input by the customer. In this case, feedback is a necessary feature and capability of your product. Wouldn’t it be nice if all features inherently capture customer and user feedback?
SoftServe’s recommendation is to go beyond what’s needed for continuous model accuracy. Try to incorporate in your capture of user feedback whether the user gets value from your model’s insights.
4. Monetize
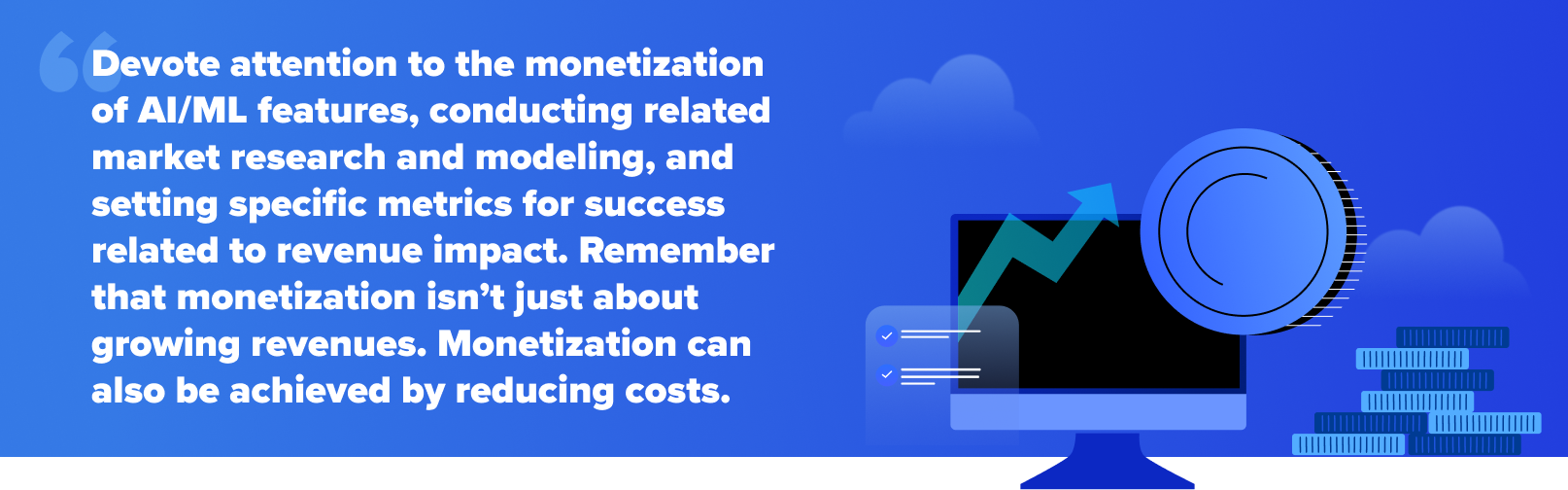
It seems this is obvious. But today, most software companies perceive AI/ML features as essential to keep pace with their competition and edgy startups. Therefore, too often, they are giving ML-fueled analysis away, undercharging, or (perhaps worst of all) not adequately assessing the value and impact to know what to charge.
The evaluation of impact and pricing models is tricky enough. Add to that the statistical nature of ML models, with varying comprehension of value by users, and the pricing exercise for ML product features becomes particularly challenging. This underscores the importance of educating your customers and receiving validated feedback from your users.
The bottom line is don’t assume ML feature functionality should be given away or included as a product feature at no added cost. A rule of thumb is if your users see the feature as an inherent and incremental improvement to your product’s features (for example, object recognition in images already displayed within your product), monetization isn’t necessary and can be counterproductive.
On the other hand, if the ML-powered features fall closer toward the spectrum of new capabilities (making predictions based on historical data, for example), then consider offering these features as a new and separately monetized capability or module.
Guidance and inspiration for monetization can be found in the MIT Sloan School of Business’s data monetization model. This is a model for data monetization readily adaptable to AI/ML monetization when thinking about ML-based inferences and analysis as applied data.
5. Data skills
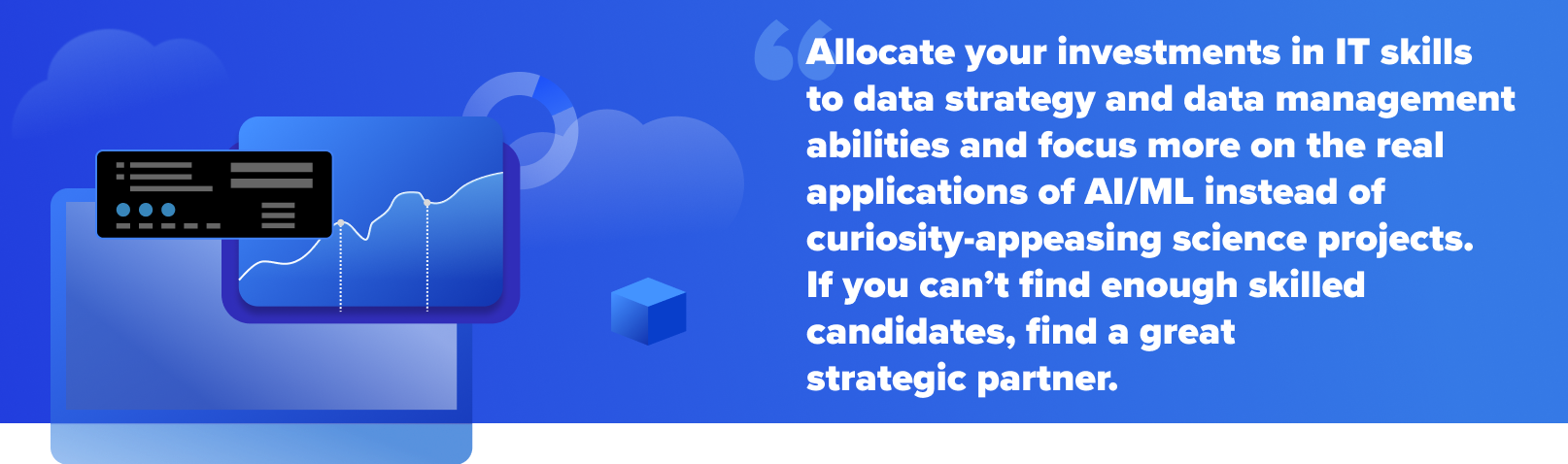
A clear gap in ML capabilities indicated by the 600 executives and technology leaders who took part in SoftServe’s research was in data analysis and data science skills. Therefore, the challenges are not in understanding and mastery of the AI/ML tools available to us today, it’s the understanding of the data and the possibilities for customer value that can be found in the data you manage.
Indeed, from public cloud vendors to emerging startups, there’s a tornado of new tool development that continues to democratize the application of AI/ML technology. Yet, those tools are dependent on the data you own and your understanding of it.
While it’s easy to say hire more data scientists and train more engineers as data scientists, the reality is it’s not easily done. Worse yet, it’s tedious and time-consuming. Current priorities must then be sacrificed in the face of never-ending pressures to deliver on your product roadmap.
The quickest route to beefing up your data science capabilities is to partner with a first-class, trusted IT services firm with a core competency in data science like SoftServe.
Beyond whatever strategy you adopt to fill your data science gap, it’s imperative your entire organization — from leadership and administration to product and operations — adopt a culture of data. Ongoing training, lunch-n-learns, seminars, webinars, and working committees, among other educational opportunities add to embracing quantitative rigor across your organization and lead to a better understanding of your data, making AI/ML capabilities possible.
6. Get serious with AI/ML now
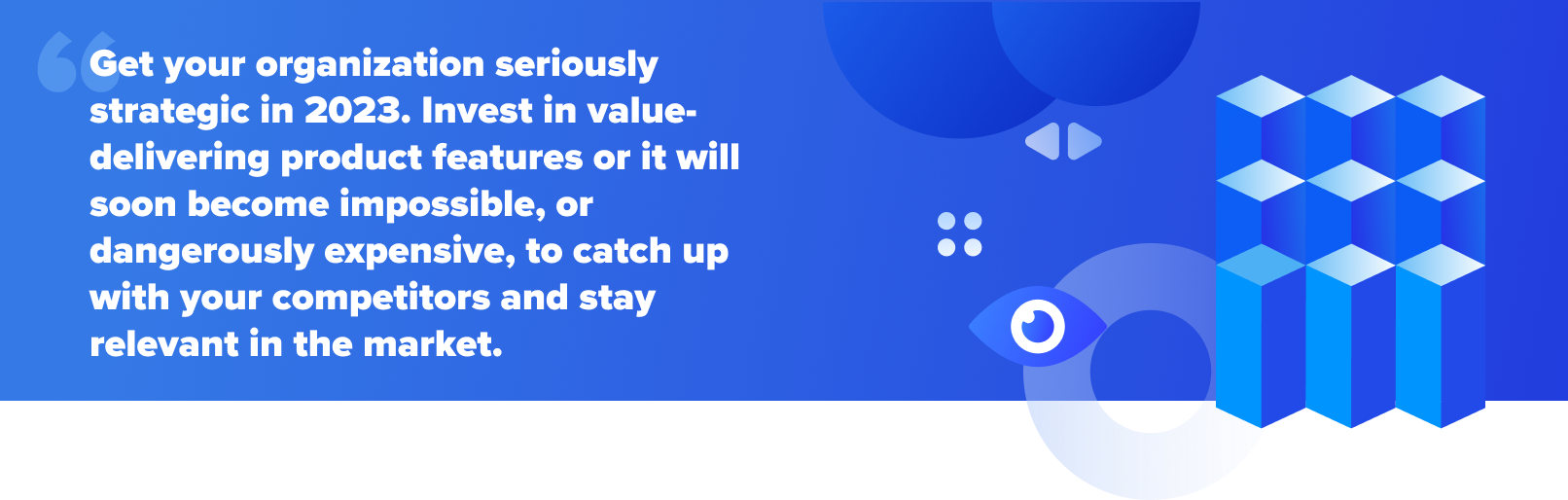
The 600 technology executives and technology leaders who took part in SoftServe’s study were overwhelmingly consistent in their belief that the viability of software companies during the next five years depends on their ability to figure out success with AI/ML technology.
The explosion of Generative AI capabilities in 2023 shows there’s truly a you-snooze-you-lose risk for most software companies, especially when you consider many of them still struggle to effectively implement first-generation AI/ML capabilities. It’s no wonder the likes of The Wall Street Journal and Harvard Business Review report it’s time to stop playing around with AI/ML technology.
In the next blog article of SoftServe’s three-part series, an interview with Iurii Milovanov, AI and Big Data Center of Excellence leader at SoftServe, we explore how software companies can get serious with AI/ML from the perspective of an AI practitioner and visionary.
The third blog article of this series features an interview with Anna Strelchuk, Product Management Center of Excellence leader at SoftServe. It explores getting serious with AI/ML from a product leader’s perspective.
You can also learn more by downloading new research, The Real State of AI/ML Capabilities in Software and Digital Native.
If you would like more information now about how SoftServe can help your organization drive product innovation and better business outcomes with AI/ML technology, let’s talk.