Curation is a well-used approach for basic media recommendation generation but, it is only the beginning of a comprehensive formula.
Genuinely personalized recommendations require inclusion of: personality trait customization, trends, social graphs, and linear consumption habits. For subscription service providers, the list extends to DVR recording data, binge watching habits, etc.
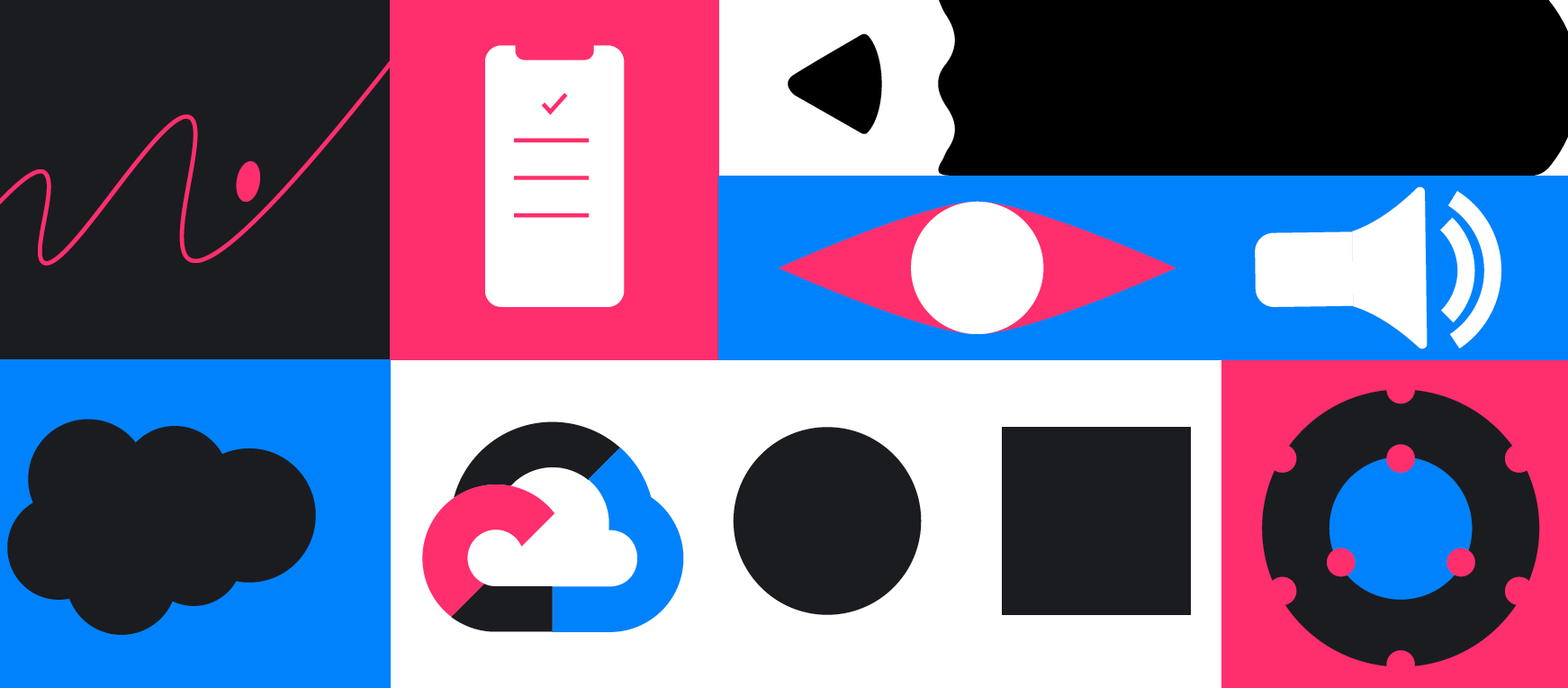
The insights from this data will allow you to deliver recommendations that go beyond what has been watched, to a trust level that encourages viewer discovery of new favorites—which, in turn, reinforces recommendation engine effectiveness and credibility.
Before an effective recommendation engine can built, however, there are a few housekeeping items that must be addressed.
First, all recommendation engines are powered by machine learning.
Machine learning is driven by artificial intelligence.
Artificial intelligence is fueled by data.
All of which should be managed on Google Cloud Platform for agility and security.
If data and cloud are not in order, then a focus on AI/ML is premature.
Once data has been consolidated, migrated, and is managed/optimized appropriately on GCP, there are a number of data points that should be included in recommendation engine development for genuine personalization.
Personality Customization
Is your viewer open, agreeable, or extroverted? Social listening insights allow you to automatically deliver recommendations that resonate—in the most tonally relatable way.
Trends
Top trends may be a great way to cast a wide net, but actual personalization requires individually-relevant trend inclusion. Regardless of how many are concerned with a trend, why include it as a recommendation to anyone who isn’t?
Social Graphs
Word of mouth has always been the undisputed champion of reach, conversion, and advocacy building. So long as it is integrated by way of invitation (transparently and securely)—integrating the recommendations of social connections (based on shared interests and favorites) can also promote increased personalization.
Linear Viewing Habits
While the rise of Netflix, Amazon, Hulu is evidence of a departure from linear viewing (especially with the younger audience), this data is still relevant, given the traditional/DTC partnerships being formed and the independent streaming offerings being rolled out to combat cord-cutting.
Integration of multiple data sources into robust, hybrid recommendation engine is not as far reaching as it might seem. This year, SoftServe (A Google Cloud Platform Premier Provider with Machine Learning Specialization) will be launching a recommendation engine built on GCP that can be integrated with existing engines or new builds.
SoftServe’s experts can help you ensure that your customer data collection and organization will drive remarkable recommendations for viewers.