In the face of growing digital native competition for audiences and ad dollars, media and entertainment companies must invest in advanced technologies to remain relevant—and profitable. Machine learning (ML) and deep learning (DL) provide consumer insights, empower personalization, and optimize customer experience. Mmedia and entertainment brands that embrace and integrate ML/DL will reap greater agility, efficiency, and sustainability.
Amazon and Netflix not only served as disruptors to the status quo, but also enablers of subsequent digital native, media startups that are not burdened with transforming legacy operations and processes. As technology progresses, ML-, and DL-empowered brands will maximize an increasing lead over those that are not.
To fully grasp these concepts, we’ll take a brief look at the definitions and relationships of these technologies to one another before reviewing the applications of ML and DL in media and entertainment (M&E).
These Russian dolls are for everyone
While the emphasis of this post is primarily on differentiating ML and DL, it must be understood that both are subsets of artificial intelligence (AI). Without AI, there can be no machine learning or deep learning. To simplify the relationship between artificial intelligence, machine learning and deep learning, one can think of them visually as technological Matryoshka (Russian) nesting dolls.
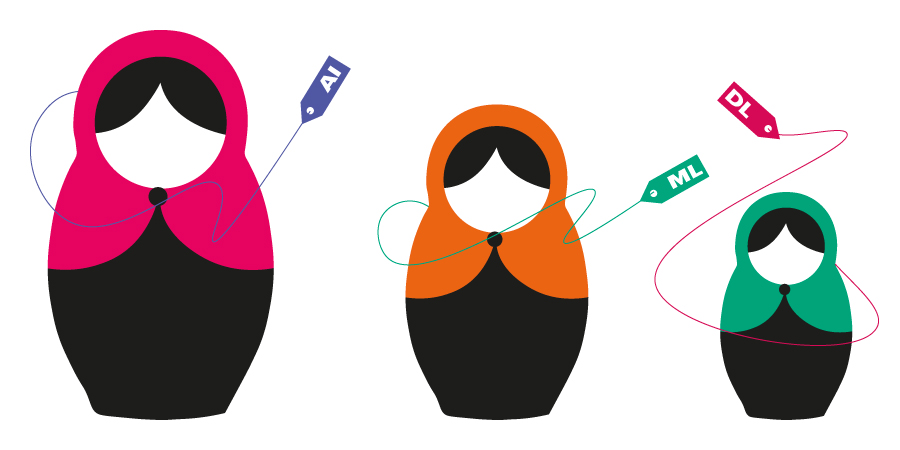
We’ll start with the largest doll (AI) and work our way inward to the mid-size doll (ML), and then finally the smallest doll (DL).
Artificial intelligence
The pursuit of defining machine-driven intelligence began more than a half-century ago. In 1956, John McCarthy—a founding pioneer of AI—established the Dartmouth Conference to accelerate serious development of artificial intelligence, stating:
“Every aspect of learning or any other feature of intelligence can in principle be so precisely described that a machine can be made to simulate it. An attempt will be made to find how to make machines use language, form abstractions and concepts, solve kinds of problems now reserved for humans, and improve themselves.”
Artificial intelligence drives the technologies that empower transformation: automation (of repetitive/error-prone processes), containerization and rendering (for scalable, on-demand content creation), and more. Artificial intelligence requires more human interaction than ML and DL because of AI’s foundational data dependency and its parental role to these subsets.
In contrast, ML and DL are learning technologies which are designed to replace repetitive and error-prone processes. So, humans develop AI to be intelligent enough to drive these automation technologies, freeing humans to be more productive in other areas such as sales, creative, or more advanced development.
To learn more about AI-driven (and cloud) applications in media and entertainment, read our latest whitepaper, THREE TECHNOLOGY MUSTS FOR M&E.
Continuing with our analogy, let’s move inward to the next two dolls, machine learning and deep learning. These two AI-driven technologies can be differentiated by nature and objective. Machine learning can be categorized as, “The objective is known” while deep learning applies to learning when the objective is unknown.
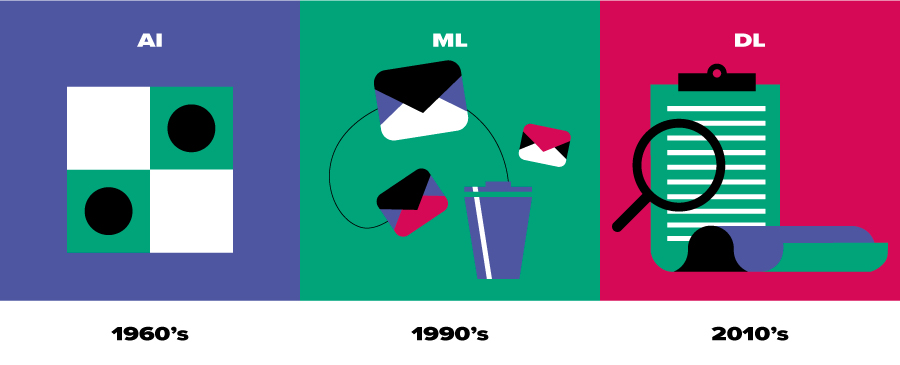
ML: “Understood, I’ll take it from here.”
Three years after McCarthy’s proclamation of his vision for AI, Arthur Samuel—a pioneer in machine learning—defined ML as, “A field of study that gives computers the ability to learn without being explicitly programmed.”
When exposed to data, ML can modify progressively, very much like a child learns to crawl and eventually walk from birth. Machine learning algorithms optimize for a known objective—either to minimize error, or to perpetually improve predictive accuracy.
DL: “Let’s find a more accurate answer—and faster.”
This subset is most closely associated to the functions of the human brain and computes vast data sets in a hierarchal manner.
Driven by graphic processing units (GPUs), DL solves more complex problems with record accuracy, and is responsible for technological advances including image and sound recognition, recommendation systems, natural language processing (NLP), and more.
Machine learning eliminates error prone, human processes that not only improve efficiency and reduce loss, but also free talent to invest time in more productive and innovative efforts. Pre-population of data in platforms, trafficking rules, and VAST tag management are areas where ML transforms efficiency. Deep learning drives the most relevant advances related to personalized user experiences through recommendation engines, chatbots, and even neuromarketing research. Read how to optimize repetitive M&E operations in our whitepaper “Stop watching screens”.
In closing
Enterprise companies that are optimizing AI, ML, and DL now will have a significant competitive advantage as these technologies evolve into industry standards of M&E infrastructure.
At SoftServe, we have a global team of data, AI, and ML/DL experts that is over 5,500 strong, with 26 years of experience, and over 4,500 enterprise success stories to date. The transformational journey to fully benefit from AI, ML, and DL is a process, but we champion thinking big, and starting small today.
Let's talk about how AI, ML, and DL will transform your company today.