SoftServe was honored to sponsor Shoptalk, one of the biggest retail conferences in the US and the world. Our best architects and analysts had the amazing chance to talk to many retail industry leaders, discussing the top challenges and issues they experience today both digitally and in general.
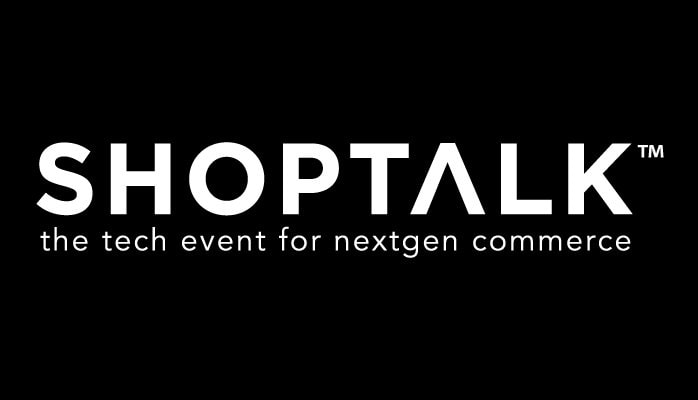
See below the top challenges—and suggested solutions—that we’ve compiled, based on feedback from retail industry experts and our own technology expertise.
Demand Prediction and Inventory Management
Challenge: Many retailers complained about unpredictable and sometimes uncontrolled inventory management. Some stores are overloaded with unwanted items, and some are suffering from lost revenue due to a lack of in-store items. Omni-channel and a combination of different delivery options add additional challenges, along with thousands of SKUs.
Retailers want to know how to forecast demand to match the items in their inventories, and to get real-time updates on when inventory is depleted for both in-store and online orders.
Solution: Modern machine learning and data integration techniques allow retailers to take back control over inventory. We advise the below steps:
- Demand prediction. Modern machine learning algorithms takes a variety of data and turns it into an advanced demand prediction model with up to 90% accuracy. Data includes in-house data, such as history of sales (PoS data), inventory history, and vendor order hi story, as well as external data such as seasonality, weather conditions and forecast, social sentiments, and event schedules, which can. For a look at this technology in action, take a look at the real-time demo that SoftServe presented at Shoptalk. The demo demonstrates a demand prediction model based on grocery store data and machine learning models we’ve created.
- Anomaly detection. Similar machine learning algorithms can keep a constant eye on sales and detect anomalies in real time for effective reaction.
- Vendor Auto Order. Implement a system that works together with PoS data and a demand prediction machine learning. This allows for the placing of automatic orders to vendors, or relocating the request for the items when the system is out of stock. Such a system accounts for the amount of time needed to relocate the item, or the time required by the vendor to send it. This system also accounts for the demand forecast data to ensure that the right items arrive when needed, neither understocking or overstocking.
- Real-time Inventory Synchronization. Inventory synchronization can be similar to relational databases: if only one item is left in-store, only one person can buy it. It can’t be bought simultaneously in-store and on-line by two different customers, even if only a few milliseconds separate these two buying requests. Inventory synchronization ensures there is no confusion between online and brick-and-mortar stores.
The Challenges of Customer Data
Problem: Many retail companies want to build a custom experience for their customers, including custom recommendations and treatments. But proper service requires precise and accurate customer data, which is not something they will necessarily want to share.
Solution: The best source of customer data is the customer. Consider the fo llowing:
- If there are problems gathering data directly from customers, retailers should be prepared to give customers something in return. Offering should include something that will make purchases less expensive, reduce friction, or lead to a better, more personalized experience. Consider offering discounts for information, or paperless systems that keep receipts and loyalty information in a central location.
- You also don’t have to ask 100% of your customers for 100% of their information. Modern machine learning techniques such as clusterization, regression models, and k-means allow the extrapolation of precisely gathered data from 30% of your customers and applying it to the remaining 70% with up to 80-90% accuracy. Combine direct information from your customers and finding similar customers based on their history, biorhythms, feedback, and demographic information.
- Explore another demo that SoftServe demoed at Shoptalk, which demonstrates cutting-edge personalization based on machine learning and the OCEAN paradigm.
Product Search and Attribution
Challenge: If a customer can’t find a product in-store, or if there are too many products to sift through in an online interface, considerable time is wasted on attribution. And if customers can’t find the products—they can’t buy them.
Solution: For e-commerce, search functions are usually executed in one of three ways: by drilling down through categories; by using texts or voice search; or by using product, similar items or “buy together” recommendations. Text search is very dependent on the product description and attributes, but keeping and curating product descriptions and attributes can take ages and considerable overhead for retailers with a large amount of stock. Make a better search function with the following:
- Automating the process of product desc riptions and attribution as much as possible to ensure customers can always easily find your items online, and to ensure minimum time to e-commerce for new items. See how a product attribution and automated machine learning algorithm works for product recommendations, based purely on the product image, in another demo SoftServe created for Shoptalk.
- For brick-and-mortar stores, specifically, we recommend using the attribution and product recommendations technology described above, as well as interactive mobile applications. These combine online search and in-store navigation capabilities, as well as customer experience and personalization technologies like picking size and color, augmented reality to check the overall look, compatibility, and more.
Interested to learn more about innovating retail? Check out our white paper, “Big Data and Psychometrics: The Threshold of Change in Retail.”