The Rise of Digital Twins
Since Marc Andreessen coined the catchphrase “Software is eating the world” in 2011, we witnessed several tectonic shifts in the digital world that set the stage for the next decade and beyond.
First, cloud has become dominant over on-prem solutions, especially for processing and analysis of big data workloads. This shift has enabled their customers with richer functionality at a faster pace and at a lower cost.
Secondly, advancements in artificial intelligence (AI), particularly in the field of deep learning, enabled automation of complex scenarios previously attainable only by humans. Although general AI remains an aspiration, specialized applications already fill a large part of our lives.
The third shift builds on the previous two and is gaining momentum. It is the virtualization of physical assets, environments, and processes that are cornerstones of the real economy—in manufacturing, transportation, energy, mining, life sciences, and many other sectors. It’s software and AI that are now eating up production lines, machines, and chemical reactions by representing them through digits and pushing to the new frontier in autonomy and optimization.
The leitmotif of this trend is quite straightforward: businesses can achieve much more in terms of productivity and innovation when their physical assets and processes are represented through digital forms (aka digital twins). Previously expensive and available only to space programs at NASA, now digital twins, powered by modern technologies, promise a giant leap for a reasonable cost.
DIGITAL TWIN TYPES
Areas of digital twin (DT) applications are boundless and are leveraged continuously to simplify asset management, maintenance, education, and communication processes by replicating accurate twins of the on-site equipment/spaces. Below, you can see a taxonomy about what a digital twin is, its key aspects with a common vocabulary across the teams. You can also learn where it can be applied, and why.
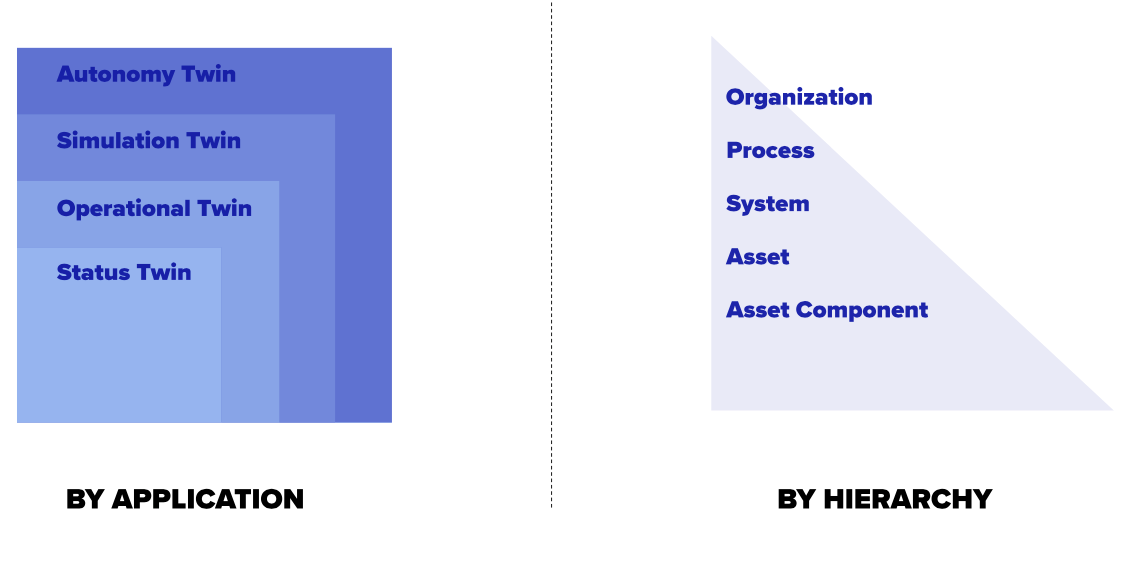
As you can see, DTs can be distinguished by their applications and hierarchy. Various combinations of those define the types of digital twins. Let’s take a look at a few real-life examples.
Example 1. Digital Twins in Industrial Context
The life of any electric motor starts from its design. Engineers create a prototype through physical modeling to test its parameters through simulation— we call it the Simulation Twin of a Future Asset (an electric motor). Once the motor is produced and installed, we need to monitor its status. To achieve it, the motor sensors read torque, voltage, temperature, vibration, and other measures to be linked to a dashboard or a 3D visual representation of the motor. It represents the Status Twin of a physical instance.
Throughout this process, sensor-generated data is collected from a motor or even many motors of the same type. For this reason, it is possible to build a model that predicts failures and fosters OEE (Overall Equipment Effectiveness) by reducing downtime through predictive maintenance. We can call it the Operational Twin of an Asset (an electric motor).
Now, let’s assume that the motor is a part of a larger industrial system that autonomously executes certain actions. In such a case, it constitutes the Autonomy Twin at the System hierarchical level.
Example 2. Supply Chain
Every digital supply chain initiative starts from collecting bits of information about supply, inventory, production, orders, and consumers to get real-time visibility of an actual supply chain and to forecast supply chain dynamics. Such data foundation represented through dashboards and forecasting models define Status and Operation Twins of a supply chain at the Organization or Process level. On the other hand, Simulation Twin typically offers simulation and optimization models to perform what-if analysis, mitigate risks, or suggest the best supplier based on cost, time-to-market, or other criteria.
The more complex scenario is, the more you can benefit from leveraging digital twins.
DIGITAL TWIN SPECTRUM
Here is an extended view on digital twin taxonomy to help match industrial needs with appropriate solutions. It is represented as an evolutionary step-by-step approach from foundational to more complex scenarios that can generate even more value:
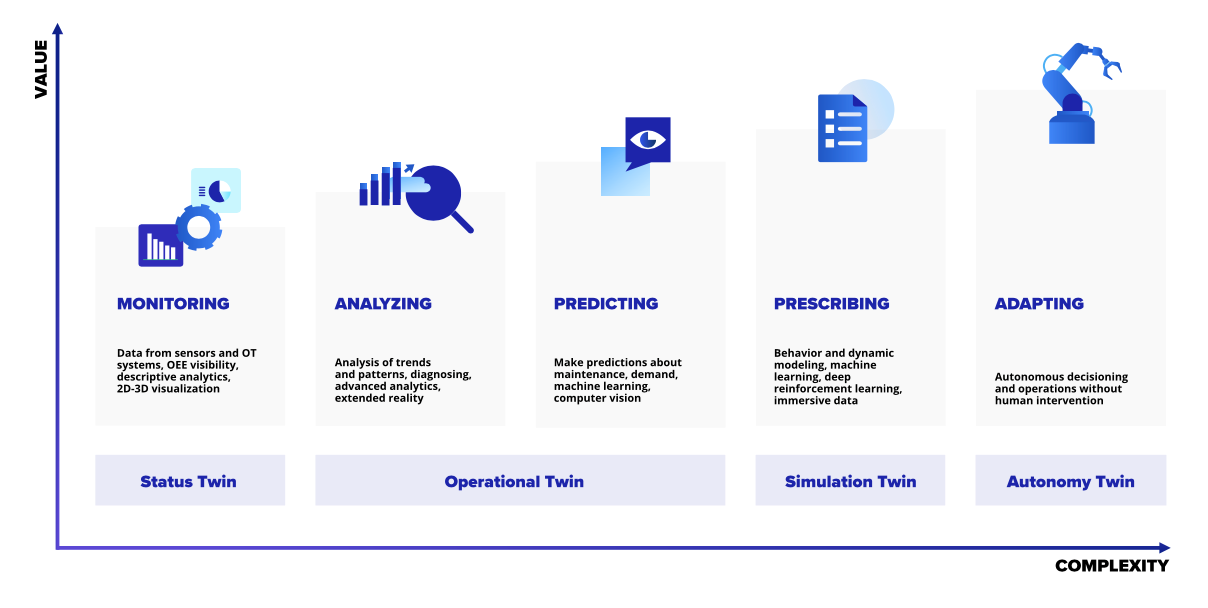
Monitoring
Real-time data collection from sensors and other sources is the first step to building operational intelligence and providing visibility into a specific process, system, or asset state. Insightful metrics and KPIs are delivered through an alerting system, dashboards, 2D-3D visual models of the physical world (immersive data) and consumed by different business functions from field engineers and supervisors to top managers who need to react to the changes in real or near real-time.
Analyzing
Insights and hidden patterns extracted using built-in machine learning and self-service analytics foster awareness and accelerate root-cause analysis. Operational digital twins provide a better understanding of failure reasons or link different correlated events at the system or process level, highlighting process bottlenecks or areas for OEE improvement.
Predicting
Collected historical data along with AI/ML enable predictive analytics, thus DT can also recommend next actions or predict future events. Predictive maintenance and visual inspection are some of the examples in Industry 4.0. Demand forecasting is one of the common examples as well.
Prescribing
Modeling and simulation of new products or processes typically is the first step in the value chain for manufacturing, mining, energy, healthcare and life sciences (HCLS), for example. There is a vast selection of tools that help create DT with physical or ML models. Also, this type of DT can be used for optimization purposes. It enables what-if scenarios simulation and can find optimal operational parameters.
Adapting
The Industry 4.0 maturity index defines adaptability as the goal of digital transformation. It is achieved when a company can use collected data to make decisions that have the best possible results in the shortest time and to implement the corresponding actions automatically, without human assistance. This type of DT is vastly represented in robotics.
The table below illustrates main differences between the digital twin types and highlights key business objectives for each:
Types of Digital Twins
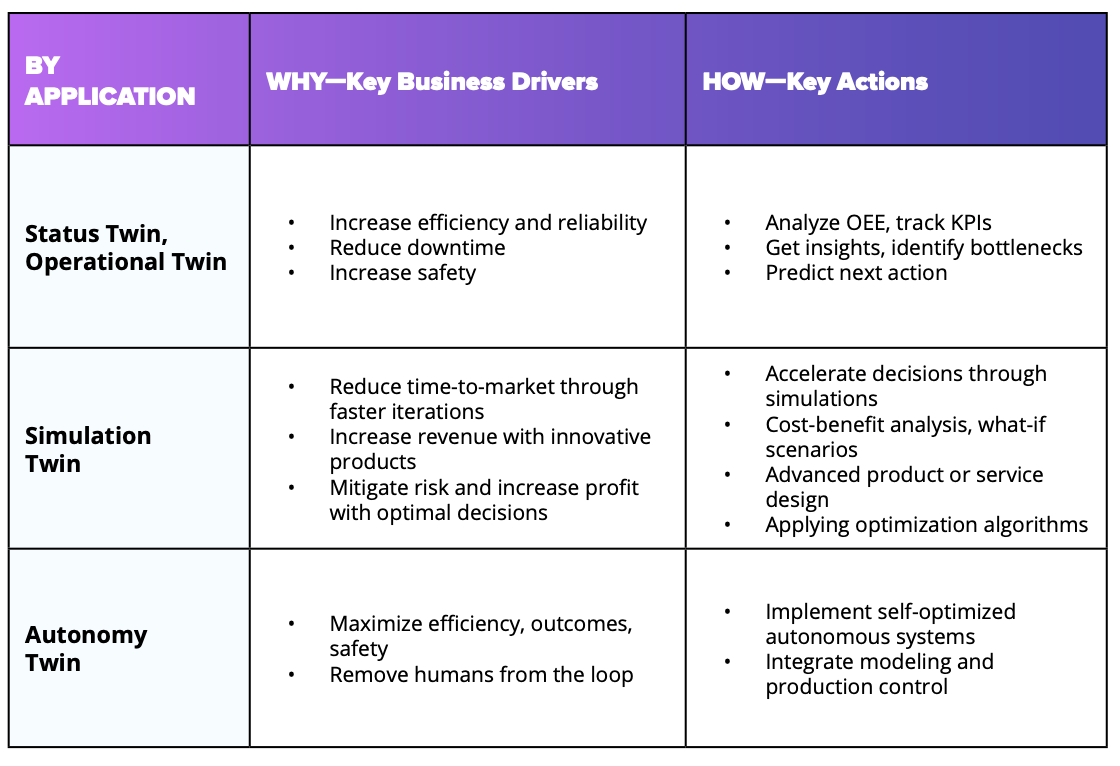
LET’S TALK about how SoftServe's guidance with digital twins will seize new opportunities to accelerate your business productivity and automation.